Using generative AI for corporate communications
Perspective from our CTO: Rutger van Bergen
Generative AI, exemplified by models like ChatGPT, Dall-E2, and GitHub Copilot, has garnered significant attention for its potential impact. This post delves into the considerations of using generative AI in corporate communications. It highlights the challenges of maintaining authenticity and tone of voice, the need for transparency regarding AI-generated content, the risk of hallucinations in generated text, and the implications of feeding data to AI models. Despite these challenges, it acknowledges that there are sensible scenarios where generative AI can be beneficial, such as proofreading, summarizing, and providing creative input. It also introduces the concept of private generative AI, which uses organization-specific data but comes with its own set of complexities.
Introduction
It is undeniable that in the past year or so, a lot of attention has been paid to the capabilities and potential of generative AI. This has been triggered in no small part by the public release of ChatGPT in November 2022, a Large Language Model (LLM) capable of generating what I will now refer to as “convincing text” - something we will get back to a little later. The focus on generative AI has been amplified further by other generative AI models, including the likes of Dall-E2 for images and GitHub Copilot for programming source code.
The capabilities of these services and the AI models behind them are objectively impressive. On that basis, a lot of content has been created about the way generative AI can, or in the opinion of some will inevitably, be a game-changing development in many markets and professions.
This article aims to provide my perspective on the considerations of using generative AI in corporate communications. I will focus mainly on public AI services and models for generating text that are now readily available and heavily publicized about. However, the core points can be projected onto other types of content as well. Also, in closing I will make a few comments about an upcoming development that is worth keeping an eye on.
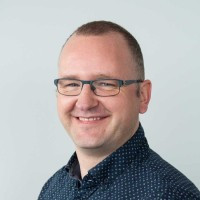
This article provides my perspective on the considerations of using generative AI in corporate communications. By leveraging its strengths while upholding the principles of authenticity, transparency, and accuracy, organizations can harness the power of generative AI to enhance their communications effectively.
Authenticity and tone of voice
For many brands and organizations, it is important that the content created, published and distributed by corporate communication teams is an authentic representation of the organization’s principles, values and, if you will, tone of voice. Although LLMs like ChatGPT are powerful AI models with a thorough grasp on the language(s) they generate content in, they currently do not embed a comprehension of human concepts like principles and values.
If generative AI is used as a primary tool for creating the content output of corporate communication, these aspects are not guarded by the model. Furthermore, LLMs generate language, which inevitably has a tone of voice. And although generative models can often be asked to change the “mood” of their output, capturing the exact tone of voice that connects with an organization’s or brand’s identity is, I would say, an unreasonable ask of the LLM used.
Transparency
Somewhat related to the point of authenticity, it’s relevant that there may soon be legal requirements concerning transparency about the use of generative AI for creating content. For one, the AI Act that is currently under development within the European Union is likely to stipulate that AI-generated content must be accompanied by clear disclosure that generative AI was indeed used. One could argue that such a disclosure could “taint” the authenticity of content it accompanies.
Other clauses in aforementioned legislation are likely to state that overviews must be provided of copyrighted data that was used to train the model. This can lead to undesirable situations if such materials include those of sources a brand or organization would not want to associate with.
Hallucinations
Generative AI services based on LLMs are built and trained to generate language that is received positively by their users. In achieving that goal, which can be paraphrased as “not wanting to sell a ‘no’”, generative AI has become great at creating content that is convincing. However, that is not the same as it being accurate.
The fact that generative AI can “make up” things to fill gaps its responses would otherwise contain - gaps that could “disappoint” the user, which is something the models are trained to prevent - is a well-known characteristic of generative AI called “hallucination”. Hallucination being an inherent characteristic of LLM-based generative AI, every apparent fact in content created by generative AI effectively needs to be fact checked by a human.
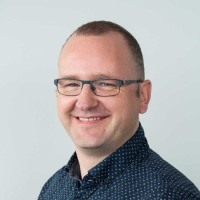
The use of private generative AI could most likely address some of the concerns mentioned above, at least in part. As generative AI continues to find its place within the landscape of corporate communications, it's essential for organizations to strike a balance between harnessing its capabilities and addressing its limitations. I’d say it’s a technological development that is worth keeping an eye on.
Feeding the model
The models backing generative AI services are trained on vast amounts of data. At the moment of first release of a generative AI service, data sources used for training tend to be selected data sets that include publicly available information on the Internet - although the exact composition of training data is effectively unclear.
What is generally true is that after initial release, the conversations with the generative AI service are themselves used to further train the model by default. Certain providers do allow this to be switched off, usually at the expense of certain functionality like retention of conversation history.
As a general rule of thumb, anything that is shared with an AI service on which model training has not been disabled should be considered to be out there in the public domain. Particularly in the case of future, embargoed communications this is very important to consider.
Sensible scenarios
Considering the previous, it may seem that I’m making the point that no sensible scenarios for using generative AI exist in the context of corporate communications. This is not the case. The capabilities that generative AI models have in the area of human language can also be used to process content that is originally written by humans.
Examples of scenarios in which this capability of LLM-based AI services can safely be used:
- Asking for a review of a human-written text, to identify mistakes in grammar, obvious inconsistencies or “gaps” in the content.
- Extracting summaries or lists of key points/topics.
- Suggesting titles, ideas, etc.
In a slightly less “strictly phrased” sense, generative AI can also be used to “unblock” a human writing session that gets stuck due to, let’s say, a dip in inspiration. As in: providing it with a manually written text that is known to be incomplete, and asking for suggestions on how to add a missing topic or perspective.
It remains important to primarily use the actual, textual output of generative AI services as thought starters, feedback or suggestions for improvement, not as content that should be copied “blindly”. And of course, in all cases where generative AI is used the points made in previous sections - and those under “Feeding the model” in particular - still need to be considered.
Upcoming: private generative AI
Up to this point, I have focused on the use of public generative AI services that are readily available. A type of generative AI that is currently under active development and seeing initial adoption is based on so-called “private generative AI”. This is a form of generative AI that’s trained with private data sets, those being data sets owned by the organization operating the AI model.
The use of private generative AI could most likely address some of the concerns mentioned above, at least in part. At the same time, it comes with its own caveats.
For one, a private AI implementation needs to be trained with sufficiently large, correct and balanced data sets. These data sets need to be collected, vetted and composed, which requires specific expertise.
Also, a generative AI model - public or private - will generate output based on the data it was trained on. Public generative AI services are so “universally powerful” in part because their training data sets are very large and diverse. Private AI will almost invariably be trained on much smaller and more focused data sets, leading to output one could describe somewhat disrespectfully as “variations of the same”.
Although this can be perfectly fine for certain applications, corporate communication often relates to new developments, at least from the perspective of the organization in question. This means that private data sets “documenting the past” may not project too well into the future - at least from the perspective of the actual content that is generated.
The use of private generative AI is a more recent development, and some of the aspects mentioned here may be ironed out over time.
Conclusion
As generative AI continues to find its place within the landscape of corporate communications, it's essential for organizations to strike a balance between harnessing its capabilities and addressing its limitations. The emergence of private generative AI offers promise in mitigating some concerns, yet the challenges of data quality and diversity remain. As we navigate this evolving landscape, it is crucial to view generative AI as a tool for augmentation rather than a replacement for human creativity and judgment. By leveraging its strengths while upholding the principles of authenticity, transparency, and accuracy, organizations can harness the power of generative AI to enhance their communications effectively. As such, I’d say it’s a technological development that is worth keeping an eye on.